As in real estate, so in cell biology: location is key. Knowing where a protein localizes in a cell gives insight into its function, and new research published in G3 describes a method to accurately identify a protein’s subcellular localization through high-throughput microscopy and machine learning.
To determine a protein’s subcellular localization, researchers can tag an endogenous protein with a fluorescent marker, image cells for the fluorescent signal, and analyze the image to determine in which cellular compartment the protein resides based on the characteristic shapes of those compartments. To do this by hand would be an impossibility, but thanks to advances in high-throughput microscopy, high-quality, rich imaging data is easy to generate. The remaining challenge is the production of accurate, automated analyses of these images—that’s where machine learning steps in. Machine learning is a process by which a program is not explicitly coded to perform its function but can learn to do so through training.
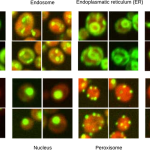
Figure from Pärnamaa and Parts, 2017. Original images from Chong et al., 2015.
In the May issue of G3, Pärnamaa and Parts present a workflow that enables assignment of a tagged protein to a subcellular compartment with 91% accuracy. They used a technique called deep learning, which combines the use of neural networks—collections of simple computational units that interact in a manner inspired by the human brain—with the associated machine learning techniques. The authors created a neural network called DeepYeast and trained the network on a large-scale data set derived from proteome-level, high-throughput microscopy images of yeast cells (Chong et al., 2015). DeepYeast was able to classify the subcellular localization of proteins in similar datasets, assigning each tagged protein to one of twelve subcellular compartments. This system gave the most accurate localizations of any similar study to date, and it serves as a robust example of the usefulness of deep learning in analyzing high-throughput microscopy data. Very large microscopy datasets are being generated more rapidly than ever, thanks to recent technological advances, and Pärnamaa and Parts demonstrate that machine learning can help solve the substantial problem of analyzing such rich datasets.
CITATIONS
Pärnamaa, T., and Parts, L. 2017. Accurate Classification of Protein Subcellular Localization from High-Throughput Microscopy Images Using Deep Learning. G3, 7(5): 1385-1392; doi: 10.1534/g3.116.033654 http://www.g3journal.org/content/7/5/1385
Chong, Y.T., Koh, J.L.Y., Friesen, H., Duffy, S.K., Cox, M.J., Moses, A., Moffat, J., Boone, C., Andrews, B.J. 2015. Yeast Proteome Dynamics from Single Cell Imaging and Automated Analysis. Cell 161(6): 1413-1424; doi: 10.1016/j.cell.2015.04.051 http://www.cell.com/cell/fulltext/S0092-8674(15)00526-7