A landmark paper published in GENETICS founded the field of genomic prediction before the requisite technology was available.
When a new technology is developed, it can allow scientists to make great strides in addressing longstanding questions. Occasionally, however, researchers think so critically about a knowledge gap in their field that they’re able to propose a new methodology that anticipates the technology needed to make it a reality.
This is precisely what Theo Meuwissen, Ben Hayes, and Mike Goddard accomplished with their 2001 paper Prediction of Total Genetic Value Using Genome-Wide Dense Marker Maps. In it, they laid out a framework for predicting breeding values from genome-wide marker information, using simulated data to compare different approaches. The catch? There wasn’t a way to do what they were proposing—the technology didn’t exist yet.
Despite this seemingly major drawback, the authors were able to successfully use theory and simulated data to propose methods that would one day prove to revolutionize animal breeding strategies.
“In retrospect, the paper was a bit of a thought piece,” says Hayes. “Imagine if we could do this: what would it look like?”
The central goal of selective animal and plant breeding is increasing the genetic gain—that is, enhanced performance—of economically important traits. This was classically achieved by meticulously recording individuals’ phenotypic information in a population and using these records to estimate breeding values and select the best breeders for establishing the next generation. As the genomic era began to bloom toward the end of the 20th century, researchers began to incorporate genotype data into their selection strategies.
“The prevalent attitude was to try and map individual quantitative trait loci (QTLs) and then incorporate them into decisions about selection of animals,” according to Goddard.
But most of the traits in question were not associated with a small number of genes or markers, as originally anticipated. Instead, the relevant traits were likely controlled by many genes of small effects—hundreds or even thousands of genes, in fact. Existing methods were geared toward mutations of large effect, which the field was discovering weren’t likely to be found.
As the complexity of the genomic architecture underlying these traits was becoming clearer, genotyping technologies were becoming more advanced.
“It had been predicted that we would get dense marker data, but we didn’t know what to do with it. We were trying to figure out what to do if we were able to get dense marker data in a cost-efficient way,” says Meuwissen.
They explored a genome-wide approach to predict breeding values without mapping specific QTLs. They needed a high density of markers across the genome for this type of approach to work, but since that kind of real data didn’t exist yet, they simulated a genome and marker set and tested a number of statistical methods. After comparing linear regression, Best Linear Unbiased Prediction (BLUP), and multiple Bayesian methods (termed BayesA and BayesB), they concluded that “selection on genetic values predicted from markers could substantially increase the rate of genetic gain in animals and plants, especially if combined with reproductive techniques to shorten the generation interval.”
They published their work in GENETICS, noting presciently that “the advent of DNA chip technology may make genotyping of many animals for many of these markers feasible (and perhaps even cost effective).” But since SNP chips weren’t yet in the hands of researchers, the paper didn’t spark an immediate revolution in quantitative genetics or animal breeding. Meuwissen, Hayes, and Goddard had founded the field of genomic selection (also termed genome-wide prediction), but the full potential of their findings wouldn’t be realized for a number of years.
“The paper really sat in the cupboard until the technological advance came along,” says Hayes.
Thankfully, they didn’t have to wait too long: by the end of the decade, SNP chips—which allow simultaneous genotyping of thousands of markers—were available for major livestock species. And with the availability of SNP chips came an explosion of interest in the paper that founded genomic selection.
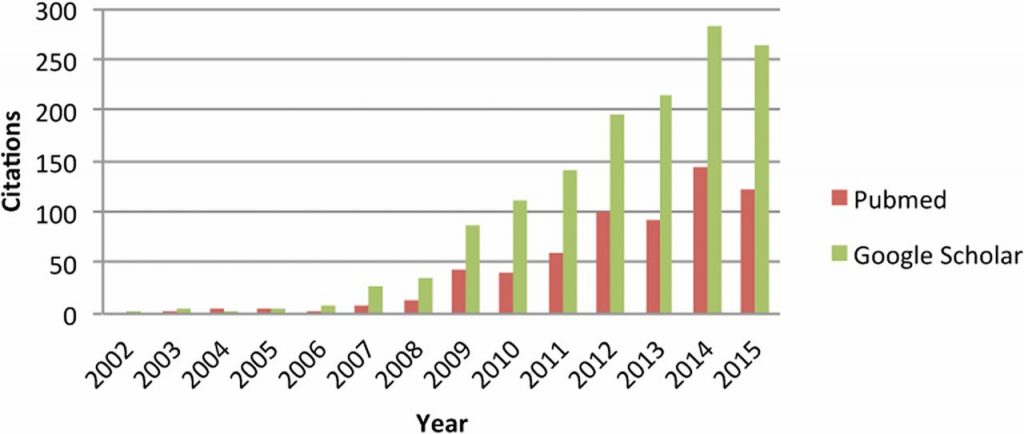
Citations to Meuwissen et al. (2001) according to PubMed and Google Scholar. From de Koning (2016).
In the nearly two decades since, the field has grown and changed in a variety of ways. For one, genotyping technology has continued to improve.
“It started off being a relatively small number of SNPs (~10,000 on the first bovine chip), and now you can get 600,000. SNP tech came onstream and rapidly advanced,” notes Goddard.
Additionally, these methodologies have also been applied more widely than livestock breeding—most notably to plant breeding and to human genetic studies of disease risk prediction. For more insight into the similarities and differences in how the methods are applied in different settings, see the new review published this month in GENETICS by Naomi Wray and colleagues.
What’s next for genomic prediction?
Researchers are still working on the best way to use whole genome sequencing (WGS) data instead of SNP chip data—though it’s now easier and cheaper than ever to sequence entire genomes, there hasn’t been much advantage to using WGS data over SNP data to date.
There are also challenges related to applying genomic prediction across breeds.
“Doing genomic prediction across breeds really doesn’t work well at the moment,” explains Hayes. “This is a problem because, in some breeds, it’s cost prohibitive to build the populations needed to drive genomic selection. There’s a lot of work going on about borrowing information across breeds.”
And as genomic prediction is being implemented widely and in many different species, it’s important for breeders to keep an eye on genomic diversity within their populations.
“We’re getting increasingly effective tools, but if we run out of diversity, we won’t be able to maintain the selection response we see today into the future,” notes Meuwissen.
Through the intervening years, the methods laid out in the 2001 paper have stood the test of time, with BayesB remaining at the forefront of genomic prediction. The field continues to grow and develop, moving into new species and honing the technologies—goals aided by the Genomic Prediction series launched in 2012 at the GSA Journals. Since then, GENETICS and G3 have collected an exciting body of work, encouraging the exploration of methods and the sharing of data to advance the field.
Genomic prediction is a striking demonstration of how science needn’t be limited by existing technology. In some cases, theoretical advances can even predict the future and help us make the most of technological advance.
CITATIONS
Prediction of Total Genetic Value Using Genome-Wide Dense Marker Maps
T. H. E. Meuwissen, B. J. Hayes and M. E. Goddard
GENETICS April 2001, 157 (4): 1819-1829.
http://www.genetics.org/content/157/4/1819
Meuwissen et al. on Genomic Selection
Dirk-Jan de Koning
GENETICS May 2016, 203(1): 5-7.
https://doi.org/10.1534/genetics.116.189795
http://www.genetics.org/content/203/1/5